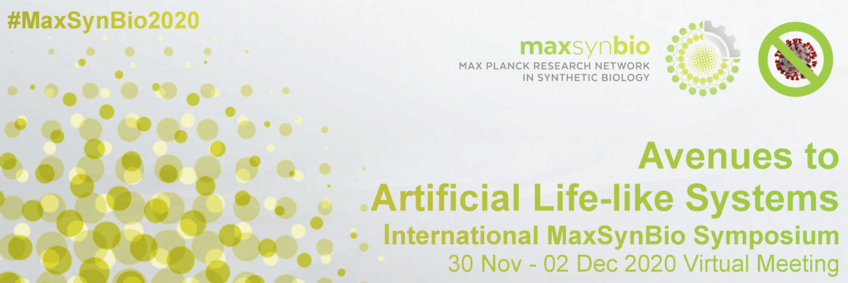
#26 - ML-guided Protein Sequence Design
Cuong To (Cinference UG, Berlin)
Tuesday, 01 Dec 21:15 - 22:00 CET
Access to the BigBlueButton rooom for this Mini Talk via the lists for Monday and Tuesday.
Please make yourself familiar with BigBlueButton before you join the Mini Talk - read the instructions.
Abstract
Title: ML-guided Protein Sequence Design
Author(s): Cuong Toa, Christian Wirschinga
Affiliation(s): aCinference UG, Berlin
Abstract: Protein sequence engineering has a wide range of applications from designing better enzymes to constructing virus capsids used in gene therapy. To solve this complex problem, biologists traditionally use directed evolution in which a set of variants are induced from the parent population of proteins. These variants are then screened for the phenotype of interest. The top-performing variants are then selected for the next round. This process is repeated until the desired proteins are discovered. Directed evolution is expensive and time-consuming but remains the standard approach until recently. With the convergence of deep generative machine learning (ML) models and high throughput screening technologies, the research of ML-guided directed evolution has intensified. Specifically, there are two steps in directed evolution that could be replaced by ML: variant screening and variant selection. By using predictive models we can virtually screen variants bypassing the complication of wet-lab experiments. However, we believe that ML-guided variant selection can provide higher impact innovation since the sequence space of protein is huge and the greedy-random search of directed evolution is most probably inefficient. In this mini talk, we propose an end-to-end platform where ML-guided search algorithms are coupled with high-throughput screening to enable faster and better sequence design, i.e. not limited to only protein. Moreover, we present an empirical study of the state of the art algorithms using transcription factor binding and AAV capsid tropism datasets. The results show that ML-guided directed evolution leads to good protein designs even when using biased predictive models.